Author: Giuseppina Schiavone
Fruitpunch AI is a private organization founded in 2018 in the Netherlands and operating globally in the software education sector. Fruitpunch AI aims at developing a challenge-based AI education platform, with the goal to educate AI engineers at scale with an ethical & sustainable mindset. Through the platform, learners can have access to free AI education, become part of a global community of AI for Good, be exposed to real-world experience. The challenges are set-up together with corporates or other type of organizations (including NGOs, SMEs and knowledge centers) seeking for AI talent and new AI solutions for real-world problems contributing to address “humanity’s greatest challenges like protecting our biodiversity, fighting climate change and improving healthcare and wellbeing“.
At the beginning of June, I found a LinkedIn post about the AI for Greener Cities Challenge, opened by Fruitpunch AI in collaboration with the JADS and DPDgroup Netherlands. I was looking for open projects in AI for Good possibly in connection to sustainability in the logistics, a focused sector for SAAC. I did not hesitate to apply; the challenge represented several opportunities for me i) to get insights into concrete use cases of logistics companies interested in measuring their socio-environmental impacts; ii) to manipulate new type of data and machine learning techniques; iii) introduce my work on transparency and accountability at SAAC to the AI for Good community.
On the 10th of June 2022 my application was accepted and I was enrolled into a 10-weeks program, which kicked-off on the 13th of June 2022. The challenge was managed by Dorian Groen from Fruitpunch AI, accompanied by Jonie Oostveen from JADS and Janne Wijnands from DPDgroup Netherlands, the latest being the main challenge stakeholder.
The general goal of the challenge was to Analyze driving datasets, specifically video from cameras mounted on vehicles, and come up with an AI-powered solution for a more sustainable city.
The challenge was launched as part of the exploratory activities in the DPD entrepreneurship program interested in i) understanding how to turn logistics data into value, for example through strategies for data monetization or data-driven green strategies; ii) exploring AI opportunities for logistics; iii) make those opportunities explicit.
More than 30 people across the globe participated to the challenge, all communications where held on slack and google.meet. We were suggested to consider the Berkley Driving Dataset BDD100K, as reference dataset and eventually look for alternative datasets. After a first round of proposals we voted on two main topics to dive in: 1) detection of vehicles and CO2 estimation, 2) detection of vegetation.
I worked on the detection of vehicles and CO2 estimation, together with an international and multidisciplinary team of 6 more people, Resham Sundar (junior deep learning engineer, specialized in smart mobility), Shail Chachra (senior deep learning engineer, specialized in computer vision), Shubham Baid (AI engineer, specialized in edge computing) from India, Antoine Miltenburg (business developer specialized in impact investment fund and agro-tech), Luca Simonetti (cognitive science and artificial intelligence student) from the Netherlands, Teddy Kosciuszek (data science student) from the US.
We worked using agile scrum methodology, with weekly updates and reflections. I took the role of project owner and I helped the team to define and assign tasks, on the basis of their expertise; I coordinated the activities and monitor the project execution. I stimulated the team to focus on a clear definition of the use cases setting up meeting with Janne Wijnands from DPDgroup Netherlands, our business stakeholder, to clarify business needs, requirements and expectations. We brainstormed on few relevant use cases and define criteria to select the most relevant to work on. Criteria included business relevance and feasibility given the project timeline and the data availability.
We decided to focus on the detection of light vs heavy vehicles, from which we could define CO2 emission metrics and traffic metrics, and the detection of private vs public vehicles from which we could define traffic and public transport coverage metrics.
With this focus, my technical contributions were the definition of the conceptual data model (the workflow from data gathering, processing and modeling, to metrics, insights and visualization) and the visualization of insights into mashable maps. The deep learning/AI engineers in the team worked on the selection of an appropriate machine learning model for detection of vehicles in the video images of the Berkley Driving Dataset BDD100K, specifically created for developing multiobject tracking and segmentation algorithms. Finally, the yolov5 architecture was selected, as the best performing on our sample set compared to other deep learning architectures such as yolov7, Faster-RCNN, and ConvNext.
After our final presentation, on the 22nd of August, our work was published on Fruitpunch AI blog and posted on LinkedIn.
GET IN CONTACT if you are interested in knowing more about the project!
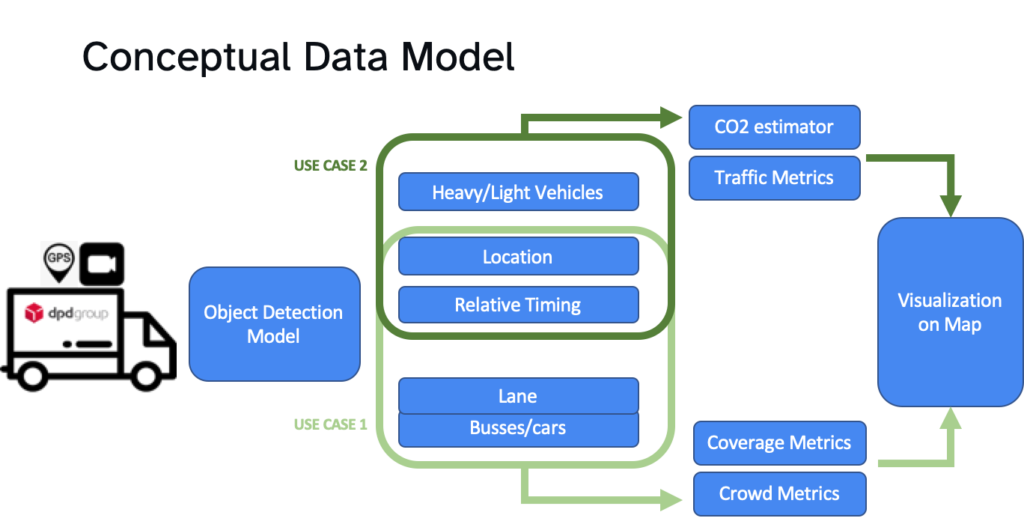
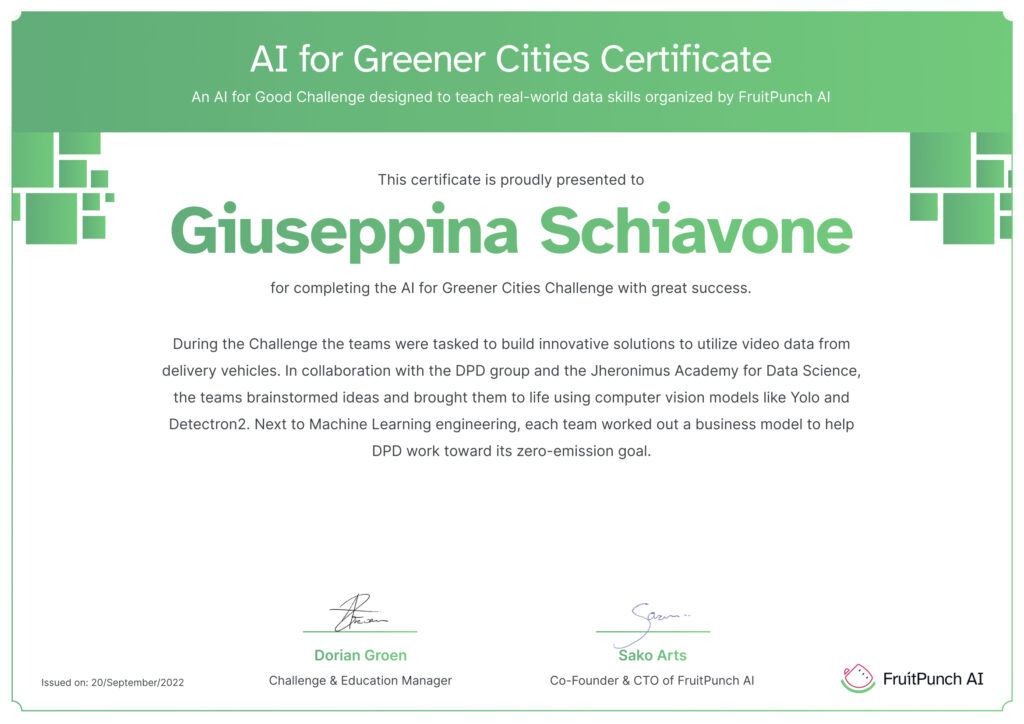
Reflections and learnings
Working on the Fruitpunch AI challenge was an interesting experience for several reasons:
- The platform: I have appreciated the value that Fruitpunch AI is bringing to the job market, beyond AI educational platform
- the platform has the potential to work as new recruitment tool, facilitating job offer – professional profile matching, for example companies can scout and evaluate AI talent through the challenges, being relatively short-time assignments
- the participation to a challenge offers opportunities to learn about a specific real-world problem, get a grasp on specific machine learning techniques, get inspired by the diverse background of the team members, share knowledge and experience,
- the platform is also a networking space and a testbed for market research, keeping in mind that promotion-like spam of own business is not encouraged
- Business relevance for SAAC: working on a the challenge with DPDgroup Netherlands as main customer has been particularly important to have a glimpse on how the leaders in parcel delivery in the Europe are looking at data exploitation for expanding their business model and for improving their sustainability strategies. I have learned that DPD Group primarily reports on 4 SDGs: SDG 8 Decent Work And Economic Growth, SDG 11 Sustainable Cities And Communities, SGD 13 Climate Actions and SDG 17 Partnerships For The Goals. Their sustainability initiatives include the air quality monitoring programme, the DPD driver App for performance monitoring and drivers motivation, the use of electric vehicles, trajectory optimization and investment in green energy for reducing CO2 emissions. Other recent initiatives and key figures of DPD Group can be found at the Sustainability Reporting 2021. I include the work performed in this challenge in the upcoming SAAC white paper “Sustainability Analytics Increases Logistics Value Beyond Delivery Services”
- Leadership skills: working on the challenge, turned out to be also relevant for me for forging further my leadership skills, specifically the ability to manage and motivating a multidisciplinary and multicultural team that could only be available part-time (most of the people participating to the challenge have full-time jobs or are full-time students), with different time-zone, and across the holiday season. In some cases, I could not even ensure my presence to meetings.
- Acknowledgements: It was an awesome experience working with my team and my special thanks go to Shail Chachra and Resham Sundar for sharing their experience on deep learning applied to object detection in video data, to Antoine Miltenburg for his business development insights and to Dorian Groen for the keeping up with the challenge management also with COVID-19.
- Way of working: The overall process thought me that even with limited time, limited resources, limited engagement some relevant results can be achieved. Having clear definition of activities and roles, together with an agile way of working, and most importantly having trust in the capacity and leadership of the team members helped to build a first proof-of-concept and to wrap up the work for the final presentation. I connect with the comments of Antoine Miltenburg relative to the importance of keeping the focus in these circumstances and to prioritize “what can be done” in place of “what is interesting to do”.
- Challenges of the challenge:
- The 10-week program officially comprises of the first 2 weeks in which masterclass training on challenge-relevant AI topics are offered, and the last 8 weeks of full immersion into finding solutions to the challenge, with a midterm and a final presentation. In our challenge, the masterclass phase was not announced and we didn’t receive any training. I consider this phase very relevant as the core of free education promoted by Fruitpunch AI and also because it addresses the fact that the participants to the challenges might have little to no knowledge about machine learning. An introductory technical masterclass could help the participants to focus on the problems or the techniques that matter.
- The challenge required a lot exploratory work, the business problem definition was open and with limited requirements, this was stimulating the thinking process from one side but was dispersing from the other side, for example causing drop-out and reducing commitment. It is understandable that 10 weeks is a short period to develop a complete project, a lot of time is invested in relationship management, because you mostly work with people that you have never met before.
- Overall: It was a very positive experience which I recommend to anyone interested in AI for good. I’m looking forward to repeat it and get involved in new interesting ESG-related challenges.